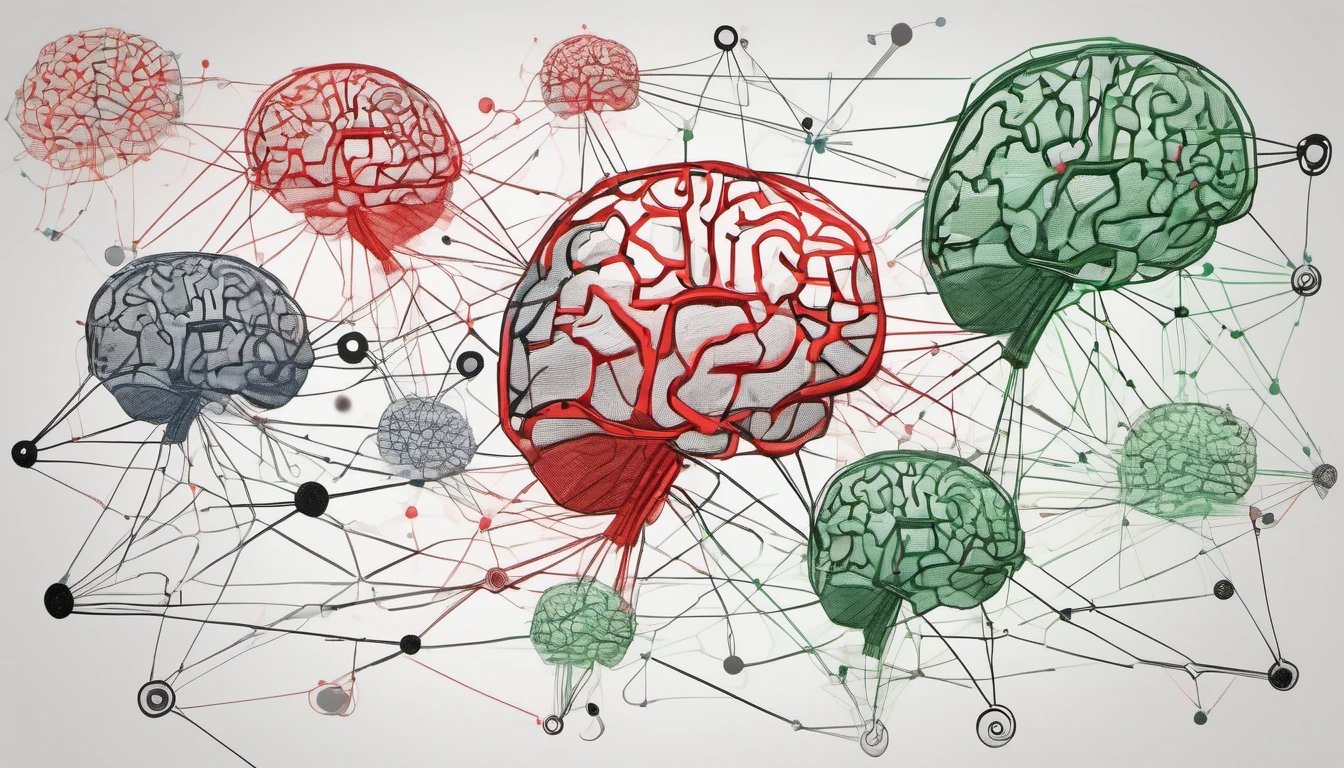
Exploring Novel Methodological Approaches for the Analysis of EEG Data: Machine Learning and Group Iterative Multiple Model Estimation
Isn't it fascinating how far we've ventured into the universe—discovering fundamental particles and reaching the moon? Yet, the depths of our own minds remain largely uncharted. EEG provides a window into the intricate workings of the brain at its operational speed. However, our statistical methods have yet to catch up. With the help of Dr. Katie Gates and Dr. Hopfinger in my undergraduate honors thesis, I embarked on a journey to bridge this gap. By harnessing modern machine learning techniques alongside the innovative GIMME algorithm, I sought to propel our understanding of the mind to new heights.
It was intriguing to find that different machine learning algorithms yield comparable results with EEG data. Therefore, I sought converging results among the algorithms. This allowed me to successfully reconcile a potential disparity between machine learning outcomes and participant behavioral results in one of the studies under investigation.
GIMME revealed distinct brain connectivity patterns between spatial attention and working memory in ERPs. Interestingly, no connectivity was observed in the alpha band for working memory, while connectivity was evident for spatial attention. These findings align with prior research on attention and memory. Notably, this pioneering application of GIMME to EEG data instills confidence in its utility for EEG research.
For this research, I was awarded highest honors from the Department of Psychology and Neuroscience and Honors Carolina.
Read my full Senior Honors Thesis here
I had the pleasure of presenting my research in the form of a poster at UNC’s Celebration of Undergraduate Research, and the Psychology and Neuroscience Departmental Honors Poster Session.